Start of the DFG-funded project (KoKAKI) to a traceable automation of design tasks in the embodiment and final design stage with artificial intelligence in May 2024
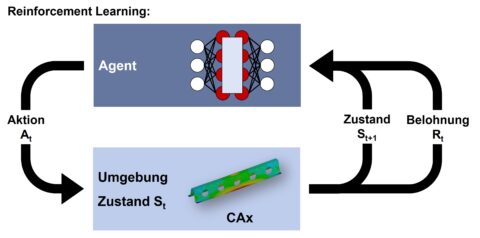
Due to cost pressure in industrialized countries and the resulting need to automate standard and routine activities, the use of AI methods is also steadily increasing in product development. Such methods are often integrated into the development process as black boxes. As a result of this implementation, the solution paths of the AI-generated solutions are difficult to understand, which can lead to incorrect conclusions. In order to make the solution path comprehensible and thus increase acceptance among product developers, the AI methods should be more closely aligned with the way product developers think. The use of reinforcement learning is a suitable method for this. The advantages of this type of machine learning are the adaptability to the mindset of the product developer and the possibility of directly testing the solution path and results.
The aim of this project is therefore to increase the traceability of automatically improved products through the application of reinforcement learning. Fiber-plastic composite components, whose design is based on complex interrelationships, are used as demonstrators for this use case.
In order to implement such an automation tool, the state description of the components should be expanded first. This ensures that the automation tool has access to the same information as the product developer. As a result, all the necessary correlations between this information can be used for the development task. Following the expansion of the state description, strategies are developed to convert intuitive exploration strategies of the product developers into exploration strategies of the AI model. Finally, possibilities are analyzed how intrinsic rewards can be used to recognize implicit relationships in the development process.